Apple Card Under Investigation by State Financial Regulator
Apple and Goldman Sachs came under fire this week after numerous users of the Apple Card, a joint venture by the two companies, took to social media claiming that the algorithm used to determine credit limits discriminated against women.
It began when Danish tech entrepreneur and racecar driver David Heinemeier Hansson wrote up an expletive-laden teardown of the card and the companies behind it after he discovered that he had access to twenty times more credit than his wife, despite the couple having filed joint tax returns. Following the twitter thread’s viral surge, other men came forward with similar stories, some noting that their wives had better credit scores than themselves.
Upon dealing with Apple’s customer service, who gave Hansson’s wife a “VIP bump” to her credit limit, raising it to match her husband’s, the entrepreneur lamented the giant’s response to his questions about the decision-making process behind Apple Card.
“Apple has handed the customer experience and their reputation as an inclusive organization over to a biased, sexist algorithm it does not understand, cannot reason with, and is unable to control,” Hansson wrote after being told by two Apple representatives that they were unable to explain the reasoning behind the inequity other than say that “it was just the algorithm.” Hansson went on later to criticize the implementations of algorithms that incorporate “biased historical training data, faulty but uncorrectable inputs, programming errors, or malicious intent” as a whole, pointing to Amazon’s recent use of an algorithmic hiring tool that taught itself to favor men.
And in a surprise twist, Apple Co-founder Steve Wozniak weighed in, saying, “The same thing happened to us. I got 10x the credit limit. We have no separate bank or credit card accounts or any separate assets. Hard to get to a human for a correction though. It’s big tech in 2019.”
Over the weekend word came from the New York Department of Financial Services that it would be investigating the practices behind the Apple Card to determine whether or not such an algorithm discriminates on the basis of sex, which is prohibited by state law in New York. This is the second such investigation recently, with the NYDFS announcing last week an investigation into the healthcare company UnitedHealth Group and its use of an algorithm that allegedly led to white patients receiving better care than black patients.
“Financial service companies are responsible for ensuring the algorithms they use do not even unintentionally discriminate against protected groups,” wrote NYDFS Superintendent Linda Lacewell in a blog post that explained the decision to investigate and called for those who believed they were affected unfairly by Apple Card to reach out. “[T]his is not just about looking into one algorithm – DFS wants to work with the tech community to make sure consumers nationwide can have confidence that the algorithms that increasingly impact their ability to access financial services do not discriminate and instead treat all individuals equally and fairly no matter their sex, color of skin, or sexual orientation.”
In their response, the Goldman Sachs Bank Support twitter account posted a note listing various factors that come into consideration when determining a person’s credit limit, asserting that they “have not and will not make decisions based on factors like gender.”
And it would appear that this is correct, at least in the literal sense, as the application process for the Apple Card does not include any questions relating to gender.
Bruce Updin of Zest AI, a company that provides machine learning software for underwriters, said of the controversy that “there’s bias in all lending models, even human lenders … race, gender, and age are built into the system. It can show up just due to the nature of the credit scoring system as FICO scores at the end of the scale can correlate to race.”
Explaining that there are connections between identity and information many humans might never perceive without machine-learning algorithms, like Nevada license plates being an indicator of the likelihood of someone’s race, Updin asserts that such links need to be weighed, balanced, and supervised by those in the banks. For Updin, transparency and explainability are the real problems here rather than the algorithms themselves.
Software exists that can pinpoint which variables are producing results that, for example, skew to prefer women over men, and can remove such factors and run the tests again, probing for differences. The trouble arises when banks find themselves unable to communicate such details for whatever reason, be it an inherent misunderstanding of their own programs or an unwillingness to explain why some of their models prefer certain groups over others.
It’s really a case of “giving up a little bit of accuracy for a lot of fairness” when choosing to remove variables that are proxies for gender, race, age, or a variety of other identifying features, according to Updin. “It’s just a lot of math, it’s not magic. The more you automate the tools, the easier it is.
“I’m convinced in 5-10 years every bank will be using machine-learning for underwriting … we don’t need to throw out the baby with the bathwater.”
Last modified: November 14, 2019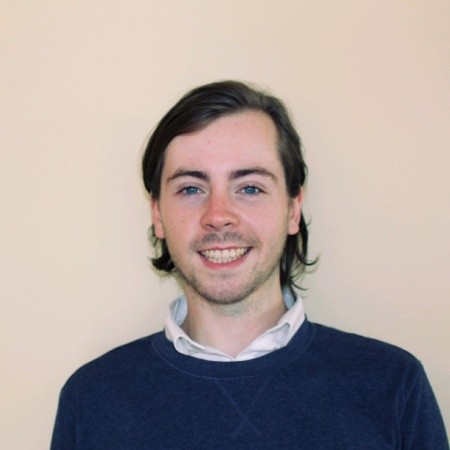
Brendan Garrett was a Reporter at deBanked.