Is Your Firm Ready for Machine Learning?
Artificial intelligence such as machine learning has the potential to dramatically shift the alternative lending and funding landscape. But humans still have a lot to learn about this budding field.
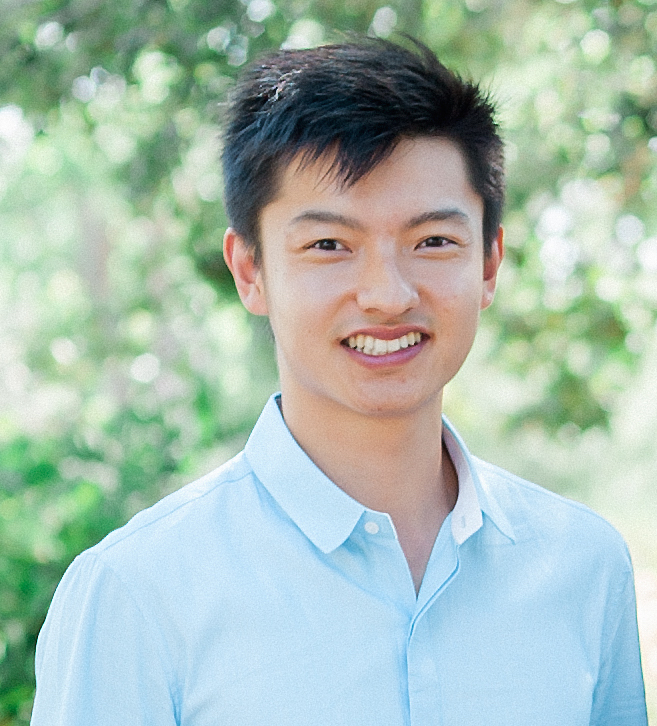
Across the industry, firms are at different points in terms of machine learning adoption. Some firms have begun to implement machine learning within underwriting in an attempt to curb fraud, get more complex insights into risk, make sounder funding decisions and achieve lower loss rates. Others are still in the R&D and planning stage, quietly laying the groundwork for future implementation across multiple areas of their business, including fraud prevention, underwriting, lead generation and collections.
“It’s entirely critical to the success of our business,” says Paul Gu, co-founder and head of product at Upstart, a consumer lending platform that uses machine learning extensively in its operations. “Done right, it completely changes the possibilities in terms of how accurate underwriting and verification are,” he says.
While there’s no absolute right way to implement machine learning within a lender’s or funder’s business, there are many data-related, regulatory and business-specific factors to consider. Because things can go very wrong from a business or regulatory perspective—or both—if machine learning is not implemented properly, firms need to be especially careful. Here are a few pointers that can help lead to a successful machine learning implementation:
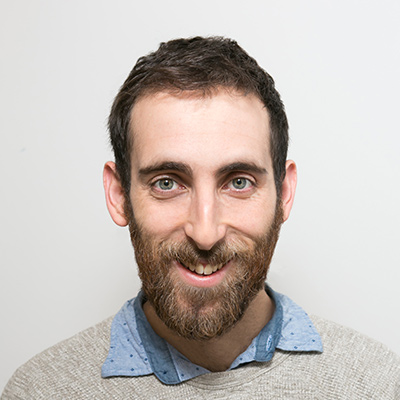
Using machine learning, funders can predict better the likelihood of default versus a rule-based model that looks at factors such as the size of the business, the size of the loan and how old the business is, for example, says Eden Amirav, co-founder and chief executive of Lending Express, a firm that relies heavily on AI to match borrowers and funders.
Machine learning takes hundreds and hundreds of parameters into account which you would never look at with a rule-based model and searches for connections. “You can find much more complex insights using these multiple data points. It’s not something a person can do,” Amirav says.
He contends that machine learning will optimize the number of small businesses that will have access to funding because it allows funders to be more precise in their risk analyses. This will open doors for some merchants who were previously turned down based on less precise models, he predicts. To help in this effort, Lending Express recently launched a new dashboard that uses AI-driven technology to help convert business loan candidates that have been previously turned down into viable applicants. The new LendingScore™ algorithm gives businesses detailed information about how they can improve different funding factors to help them unlock new funding opportunities, Amirav says.
Lenders and funders always have to be thinking about what’s next when it comes to artificial intelligence, even if they aren’t quite ready to implement it. While using machine learning for underwriting is currently the primary focus for many firms, there are many other possible use cases for the alternative lenders and funders, according to industry participants.
Lead generation and renewals are two areas that are ripe for machine learning technology, according to Paul Sitruk, chief risk officer and chief technology officer at 6th Avenue Capital, a small business funder. He predicts that it is only a matter of time before firms are using machine learning in these areas and others. “It can be applied to several areas within our existing processes,” he says.
Collection is another area where machine learning could make the process more efficient for firms. Machines can work out, based on real-life patterns, which types of customers might benefit from call reminders and which will be a waste of time for lenders, says Sandeep Bhandari, chief strategy and chief risk officer at Affirm, which uses advanced analytics to make credit decisions.
“There are different business problems that can be solved through machine learning. Lenders sometimes get too fixated on just the approve/decline problem,” he says.
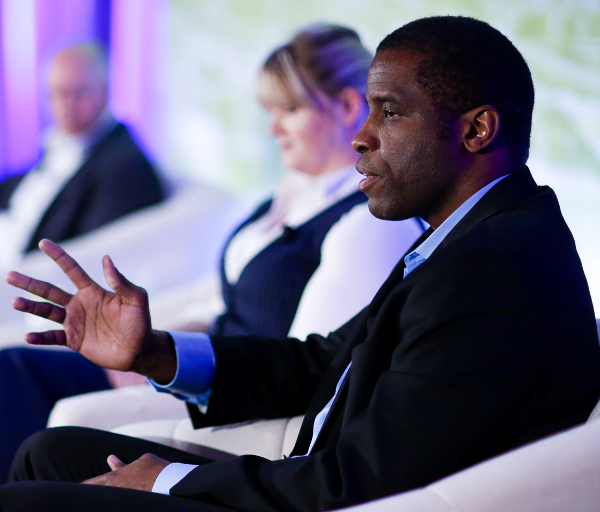
“Most underwriters don’t have enough data to effectively incorporate AI, deep learning, or machine learning tools,” says Taariq Lewis, chief executive of Aquila, a small business funder. He notes that effective research comes from the use of very large datasets that won’t fit in an excel spreadsheet for testing various hypotheses.
Problems, however, can occur when there’s too much complexity in the models and the results become too hard to understand in actionable business terms. For example, firms may use models that analyze seasonal lender performance without understanding the input assumptions, like weather impact, on certain geographies. This may lead to final results that do not make sense or are unexpected, he says.
“There’s a lot of noise in the data. There are spurious correlations. They make meaningful conclusions hard to get and hard to use,” he says.
The more precise firms can be with the data, the more predictive a machine learning model can be, says Bhandari of Affirm. So, for example, instead of looking at credit utilization ratios generally, the model might be more predictive if it includes the utilization rate over recent months in conjunction with debt balance. It’s critical to include as targeted and complete data as possible. “That’s where some of our competitive advantages come in,” Bhandari says.
Underwriters also have to pay particularly close attention that overfitting doesn’t occur. This happens when machines can perfectly predict data in your data set, but they don’t necessarily reflect real world patterns, says Gu of Upstart.
Keeping close tabs on the computer-driven models over time is also important. The model isn’t going to perform the same all along because the competitive environment changes, as do consumer preferences and behaviors. “You have to monitor what’s going well and what’s not going well all the time,” Bhandari says.
Certainly, as AI is integrated into financial services, state and federal regulators that oversee financial services are taking more of an interest. As such, firms dabbling with new technology have to be very careful that any models they are using don’t run afoul of federal Fair Lending Laws or state regulations.
“If you don’t address it early and you have a model that’s treating customers unfairly or differently, it could result in serious consequences,” says Tim Wieher, chief compliance officer and general counsel of CAN Capital, which is in the early stages of determining how to use AI within its business.
“AI will be transformative for the financial services industry,” he predicts, but says that doing it right takes significant advance planning. For instance, Wieher says it’s very important for firms to involve legal and compliance teams early in the process to review potential models, understand how the technology will impact the lending or funding process and identify the challenges and mitigate the risk.
To be sure, regulation around AI is still a very gray area since the technology is so new and it’s constantly evolving. Banking regulators in particular have been looking closely at the issues pertaining to AI such as its possible applications, short-comings, challenges and supervision. Because the waters are so untested, there can be validity in asking for regulatory and compliance advice before moving ahead full steam, some industry watchers say.
Upstart, for example, which uses AI extensively to price credit and automate the borrowing process, wanted buy-in from the Consumer Financial Protection Bureau to help ease the concern of its backers as well as to satisfy its own concerns about the legality of its efforts. So the firm submitted a no-action request to CFPB. The CFPB responded by issuing a no-action letter to Upstart in September 2017, allowing the company to use its model. In return, Upstart shares certain information with the CFPB regarding the loan applications it receives, how it decides which loans to approve, and how it will mitigate risk to consumers, as well as information on how its model expands access to credit for traditionally underserved populations.
The No-Action Letter is in force for three years and Upstart can seek to renew it if it chooses.
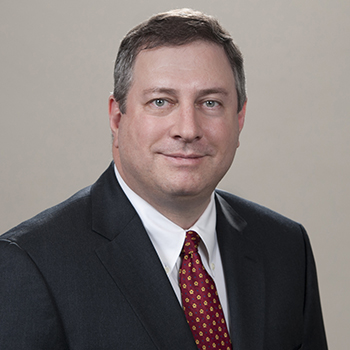
Theoretically firms could have a computer underwriting model constantly updating itself without having a human oversee what the model is doing—but it’s a bad idea, industry participants say. “I believe there are companies doing that, and it’s a risky thing to do,” says Scott M. Pearson, a partner with the law firm Ballard Spahr LLP in Los Angeles.
During review of the models—and before implementing them—people should carefully review the models and the output to make sure there’s nothing that causes intrinsic bias, says Kathryn Petralia, co-founder and president of Kabbage, which is one of the front-runners in using machine learning models to understand and predict business performance.
“If you’re not watching the machine, you don’t know how the machine is complying with regulatory requirements,” she says.
Kabbage has teams of data scientists regularly developing models that the company then reviews internally before deploying. The company is also in frequent contact with regulators about its processes. Petralia says it’s very important that firms be able to explain to regulators how their models work. “Machines aren’t very good at explaining things,” she quips.
As a best practice, Pearson of Ballard Spahr says lenders and funders shouldn’t use any machine learning model until it’s been signed off on by compliance. “That strikes a pretty good balance between getting the benefits of AI and making sure it doesn’t create a compliance problem for you,” he says.
While AI has many benefits, industry participants say alternative lenders and funders need to be mindful of how it can be applied practically and effectively within their particular business model.
Craig Focardi, senior analyst with consulting firm Celent in San Francisco, contends that the classic FICO score continues to be the gold standard for credit decisions in the U.S. He warns firms not to get overly distracted trying to find the next best thing.
“Many fintech lenders have immature risk management and operations functions. They’re better off improving those than dabbling in alternative scoring,” he says, noting that data modeling is an entirely separate core competency.
Indeed, Lewis of Aquila cautions underwriters not to view AI as a silver bullet. “AI is just one tool out of many in the lenders’ toolbox, and our industry should use it and respect its limitations,” he says.